The New Frontier - Design Considerations for AI Products
A discussion about how generative AI is reshaping product management's traditional constraints of desirability, feasibility, and viability, while exploring key design considerations like probabilistic outputs, user agency, and economic realities.
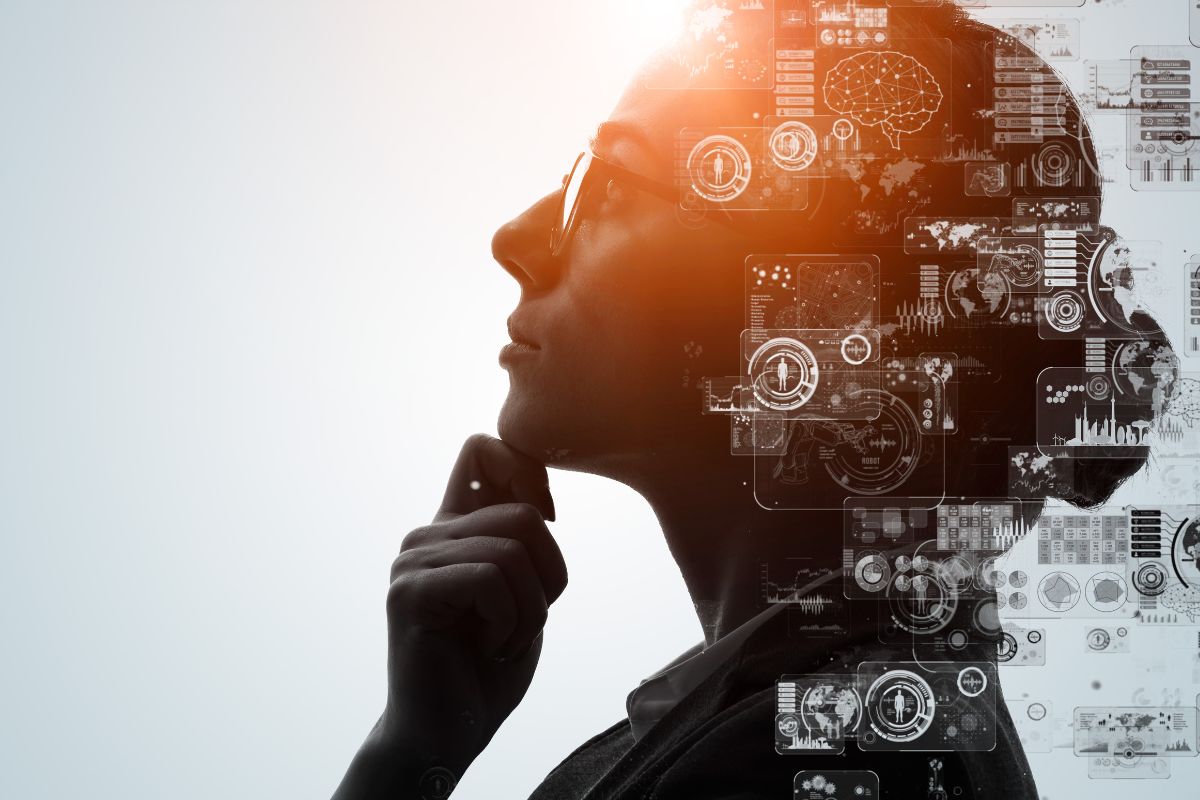
The New Frontier: Design Considerations for AI Products
The landscape of product management has undergone a seismic shift. While artificial intelligence has been part of products for years, the emergence of generative AI has fundamentally transformed how we think about AI product design. As a product manager who has witnessed this evolution, I’ve observed that we’re not just adding AI features anymore – we’re reimagining the entire product development lifecycle through an AI-first lens.
The Changing Nature of Product Management’s Triple Constraint
Product managers have long operated at the intersection of desirability, feasibility, and viability – a framework that remains relevant but has been dramatically reshaped by AI. Think of these three factors as tectonic plates that have suddenly started moving at unprecedented speeds.
Desirability has exploded beyond our traditional expectations. Just as the smartphone revolution made “there’s an app for that” a cultural touchstone, we’re now entering an era where “there’s an AI for that” is becoming the new normal. From self-driving cars to code completion, user expectations have soared to heights that would have seemed like science fiction mere years ago.
Feasibility has been redefined. Traditional software engineering operated like a recipe book – precise, deterministic, and bounded by explicit rules. AI has transformed this into something more akin to teaching a child – the system learns, adapts, and sometimes surprises us with its capabilities. Companies like NVIDIA have accelerated this transformation by making previously unthinkable computational tasks not just possible, but economically viable.
The Probabilistic Paradigm Shift
Perhaps the most profound change in AI product design is the shift from deterministic to probabilistic outputs. Imagine a traditional calculator: 2+2 always equals 4. Now, imagine asking different people to describe a sunset – you’ll get varied descriptions, all potentially valid but not identical. This is the new reality we must design for.
Consider a RAG-based (Retrieval-Augmented Generation) customer service tool. Even with temperature set to zero – a parameter that makes the model choose its most confident answer – the same question might receive slightly different responses at different times. This isn’t a bug; it’s a fundamental characteristic of AI systems that continue to learn and evolve. Setting temperature to zero is like asking someone to give their best answer – while they’ll try to be consistent, their knowledge and understanding might change over time.
The Rise of User Agency
In response to this inherent uncertainty, successful AI products have embraced a design pattern that puts users in control. Microsoft’s GitHub Copilot serves as an excellent example – it suggests code but doesn’t impose changes. This approach is like having a knowledgeable colleague looking over your shoulder: they can make suggestions, but you retain the final say.
LinkedIn has implemented this pattern beautifully in their AI-powered features. Rather than automatically generating content, they present drafts for users to review, modify, or reject. This design choice isn’t just about user experience – it’s about building trust in a world where AI outputs carry inherent uncertainty.
The Economics of AI Product Design
The miracle of AI comes with a price tag. Unlike traditional software where marginal costs approach zero, each AI interaction incurs computational costs. This economic reality has given rise to new business model patterns. Product managers must now design with resource consumption in mind, leading to tiered quota systems that balance user value with operational costs.
Think of it like electricity in your home – while it seems magical that light appears at the flip of a switch, there’s a meter running. AI products similarly need to manage this consumption, leading to innovative pricing models that align user value with resource usage.
Looking Ahead
As we navigate this new frontier, the role of AI product managers continues to evolve. We’re not just following a playbook – we’re writing it. The considerations outlined here are just the beginning. As AI capabilities expand and user expectations evolve, new design patterns will emerge.
The key to success lies in embracing the unique characteristics of AI while maintaining focus on user needs and business realities. Just as the best software products found ways to make complexity accessible, the best AI products will find ways to harness uncertainty while delivering consistent value.
What other design considerations have you encountered in AI products? How are you managing the balance between capability and predictability? The conversation is just beginning, and we all have a role in shaping its future.